January 21st, 2019 | by Jarosław Ściślak
Are AI Algorithms the Key to the Future of Technological Development?
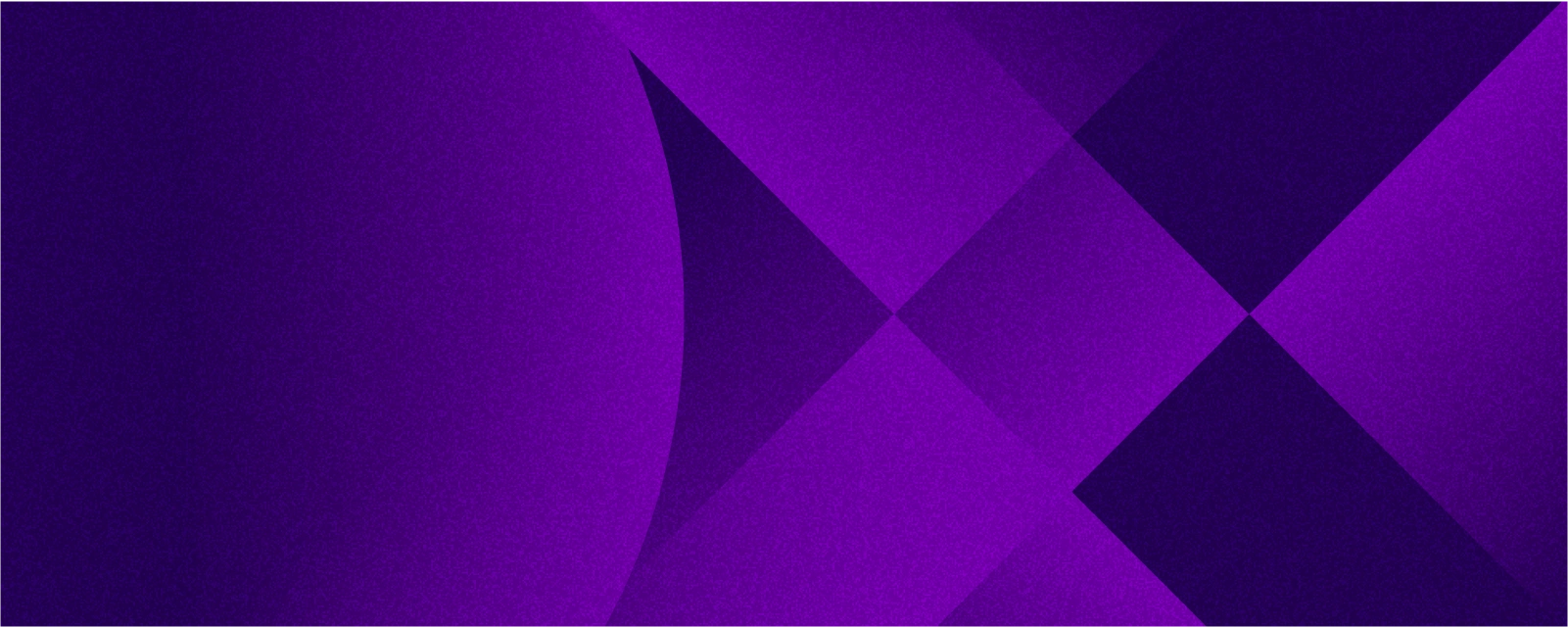
Table of contents
Very much so. Artificial intelligence (AI) is the key to further many very reliable branches of business. Not ‘promising’ but already reliable, since many solutions exist and effectively support managers today. They just need further development and polish. AI is not running on hope, though. It needs fuel – algorithms.
It’s an entirely new field of science, filled with machine learning that helps specialized algorithms in processing data and taking notes on how to deal with tasks now and in the future. With that kind of training, algorithms can improvise and react to the situation they have never encountered before. We are quickly heading into the future where discussed technology will be daily bread for many of us. Amazon Alexa and Google’s Assistant are the first strong indicators.
I’m writing this article just hours from Google’s big drop – at the morning of CES 2019 they shared the company’s big expectation with the world – Google wants to put Assistant on 1 billion devices total by the end of this month. This is an unprecedented speed, meaning all the giants are eager to push technology to the point where algorithms of artificial intelligence are ubiquitous. But what are algorithms anyway, how do they work and what can we expect from them?
The AI algorithms development
The algorithm’s goal is to learn and pull statistical findings from its experiences in order to complete built-in goals. Humans can’t predict what would be the nature of these conclusions. This means we don’t exactly know why the autonomous car will turn on the lights when it’s foggy out there. Logic suggests that when data goes into the front camera and the processing power of the chip inside the car ‘understands’ the idea of ‘I can’t see anything’, the car will turn on the lights automatically in response to the worsen conditions. Engineers teach the car to ‘think’ that way but the complete map of artificial intelligence would take billions and billions of numbers and no human can trace them to understand how the system decided on turning the lights on and not to stop the car to avoid collision. What can be understood by human beings are teaching algorithms that say how to process statistically-optimized data that is generated after every single occurrence and then incorporate it into the reality of that given autonomous car.
Read also: Bias in Artificial Intelligence (AI) – The Need for Lighting Up the ‘Black Box’
The shortest definition of an algorithm goes as follows: it is the definitive series of clearly defined actions necessary to perform previously defined tasks. The shortest definition of the place where algorithms are used is even simpler – everywhere. These formulas are like a recipe in a cookbook. From dating apps to advertisement systems, from telemetry solutions to banking systems involving FinTech and RegTech. When we buy a loaf of bread every day, an app should suggest a different kind of bread or the same on, at a different price, a block down from us. Not a roll. It should give us a notion that our preferences, habits and familiar products are important to the company buying the advertisement. It’s not about selling anything; it’s about selling what we already know to what we would like to know.
But an algorithm is not programmed to work one way nor the other. It’s all about learning, pulling statistical probability and sense from previously uploaded and autonomously generated and processed data. In the light of this, it’s easy to understand why technological giants are investing billions into technology which will generate them… even more billions. This is due to the perfectly placed technology.
Amazon’s Alexa for example uses a cloud-based voice service called Alexa Voice Services (AVS). All questions (both first and third-party) and product purchases are stored and tied to your Amazon account. The big advantage is also its open API, meaning any third-party software product developer can push its work onto Alexa. Companies like Philips or Uber are already there, stimulating the algorithm development even further.
Read also: How Automotive is Innovating the Customer Experience?
Google Assistant on the other hand, makes the most out of the giant data pool that is the Google knowledge base itself. Everything linked to the account, including purchase history and even calendar, can be browsed for answers. Algorithms are concentrated on pulling data from the huge pool and optimized so that everything makes sense when in conjunction with other company’s services. Google also assures that the data is secure and ‘protected by one of the world’s most advanced security infrastructures. Conversations in Google Home are encrypted by default’.
As says Bartłomiej Undak, a CSHARK’s board member:
Machine learning algorithms like clustering, support vector machines or neural networks have been there for decades. Mathematicians did good job by preparing a whole theoretical background but only recently we got enough technological capabilities to utilize it. Processing power, storage and data amounts have reached the critical mass and they are still growing. It is exciting to see machines figuring out their way to the solution instead of being instructed what to do. But under the hood it’s still a numbers game – no magic, just functions and parameters optimization. What makes it look “magical” is the scale at which the algorithms operate and solutions that emerge.
At CSHARK we want to make sure that by using buzz technology we don’t sacrifice privacy. We will be utilizing algorithms in the upcoming projects to ensure efficiency without violating the main principle behind the tech – it should serve people, not the other way around.
In the shadows of two great competitors, the other two are emerging. Cortana from Microsoft and Bixby from Samsung are coming in hot and with nearly 30% of consumers who uses voice assistants to voice search information about the products, market is getting awfully crowdy. Yes, according to the report by NetElixir, almost 30% of consumers who uses voice assistance features, look for information about the purchase but does not buy. Perhaps it has to do with the lack of visualization of the product and limited trust towards inability to touch the item even virtually.
These are examples of famous products, but what about some less known ones? Polish programmers developed Milla, a voice assistant for Millennium Bank. Thanks to the ASR and TTS voice modules Milla can transfer the money onto the given account just by giving her necessary number. It can also recharge the prepaid account and phone prepaid card. Milla can also remind the owner about the upcoming payment.
Intelligent systems
OK, but what about other intelligent systems, not necessarily linked to the intelligent speakers market? Do they also have a future in an intelligence-based economy?
Stefan Hartung, a board member responsible for Energy and Building Technology in Bosch, told in the recent press release, that in 10 years all electronic products will either be equipped with artificial intelligence or will be designed and manufactured with AI in mind. This is a breakthrough statement. The company wants to invest around 300 million euros to expand already existing Bosch Center of Artificial Intelligence (BCAI) by 2021. The BCAI currently employs 170 experts globally; it is planned to expand the employment to 400. Currently they have over 80 projects in software product development, from automated driving to medical applications and manufacturing. Many of them are being carried out in cooperation with academic partners, on the list are universities in Tübingen, Stuttgart, and Amsterdam.
Read also: The Future of Automotive IoT – 5G and Integration
At the Bosch campus in Renningen, the company are developing projects in 6 areas:
- Deep learning. Big data analytics with thousands of cores and almost unimaginable processing power can improve industrial processes and production methods.
- Dynamic systems modelling. Based on advanced mathematics and physics, this method is helpful when measuring dynamic properties of elements. In other words, it’s predictive maintenance that saves loads of money on parts due to information about their usage.
Read also: Predictive Software Predicts Future Events
- Robotics. The focus is put on assistive technologies that improve logistics inside industrial environments. In this scenario robots assist in maintaining the flow on assembly lines and perform similar tasks.
- Automotive. The optimization of radio and video data flowing left and right from sensors inside the autonomic cars is the challenge. Since more and more cars will be autonomous or at least packed with electronics, vehicles will be able to prevent accidents based on the behaviour of the ‘knowledge’ coming from shared data pools.
- Security of autonomous cars through deep learning algorithms. Thanks to constant analysis of the data and real-time environment conditions, the autonomic system is able to learn on its own. Without supervision and with machine learning algorithms, autonomic cars can avoid dangerous situations even if not equipped with specific scenarios.
- Industry 4.0. The enormous loads of data in modern factories are not manager’s whims. Automatic analysis of the constant data flow is to favour a flexible approach to production and management.
Read also: Automotive Retrofitting – An Old Car Is New Again
The Verge just a few days ago reported that 100 million of Alexa devices have been sold. Amazon can be satisfied, but with previously mentioned Google Assistant and their 1 billion plan, it’s a still a long way to go. Nevertheless, the battle for the easily accessible service based on the work of algorithms is in full swing. With industrial solutions on top of that, I wouldn’t be concerned about the future. Software product developers, mathematicians, machine learning specialists and product managers alike can work on new solutions. There is still a lot to be done, we are witnessing impressive beginnings.